Focus areas
Background
We take joy in mathematical optimization and its profitable application to practical problems. Numerical algorithms for optimization are now capable of solving problems that were considered completely hopeless just a decade ago. Often, these algorithms are even freely available and can achieve immediate added value with some programming experience and mathematical background knowledge. We focus on making this added value more accessible for a range of specific tasks, especially for SMEs.
Problem statement
Optimization is universally useful yet sometimes difficult to divide into subareas.
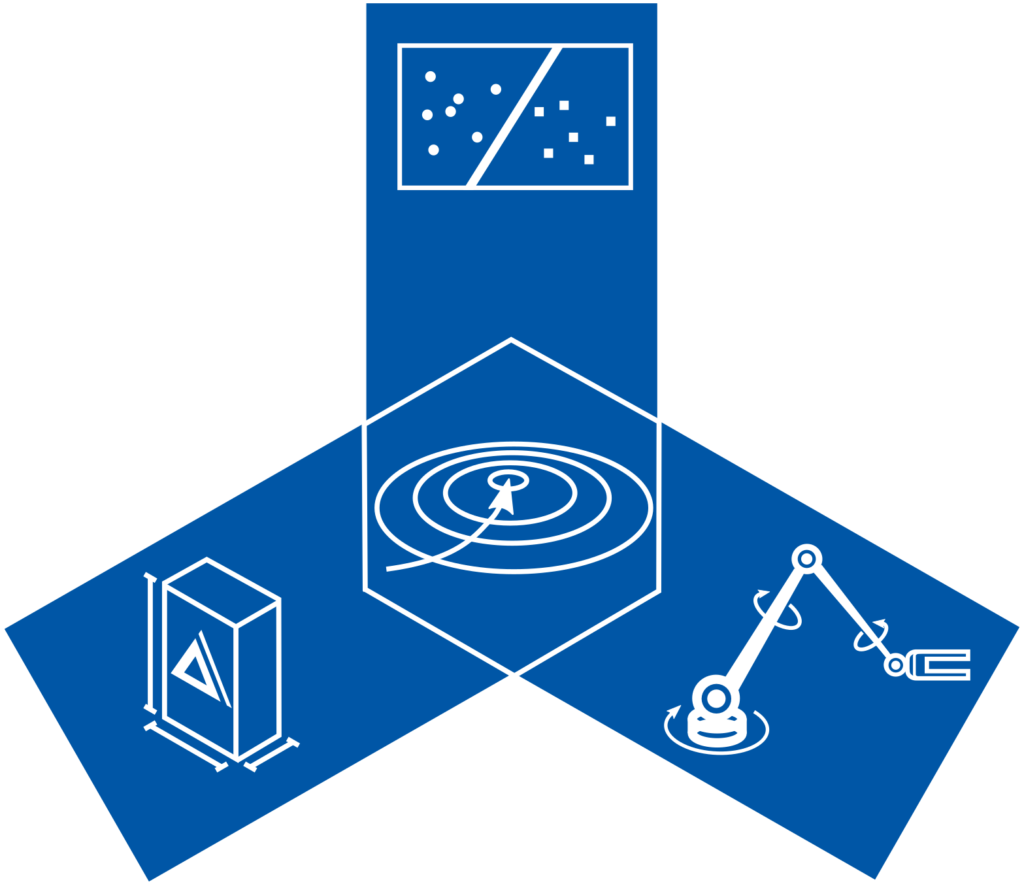
Practical applications from very different subject areas often have the same mathematical formulation. And sometimes, slightly different tasks from the same area require fundamentally different approaches.
We specialize in optimal decisions under uncertainty. This aspect is particularly prominent in the evaluation of measurement data. But not only there — with optimization, we solve problems from the following areas:
- Optimal estimation: Evaluation of measurement data, parameter estimation, quantification of uncertainties.
- Optimal design: Design of schedules, material flows in networks, design of experiments and series of experiments.
- Optimal control : Stabilization of time-varying systems, adaptive logistics decisions, reinforcement learning.
Competencies
You are in the right place if you have a problem that requires mathematical modeling and for which a quality measure can be established. This problem should be solvable through software integrated into the business process or individual mathematical analyses. If you are unsure about any of the points mentioned above, just ask us!